AI for Fraud Detection in Insurance: Advancements and Implications
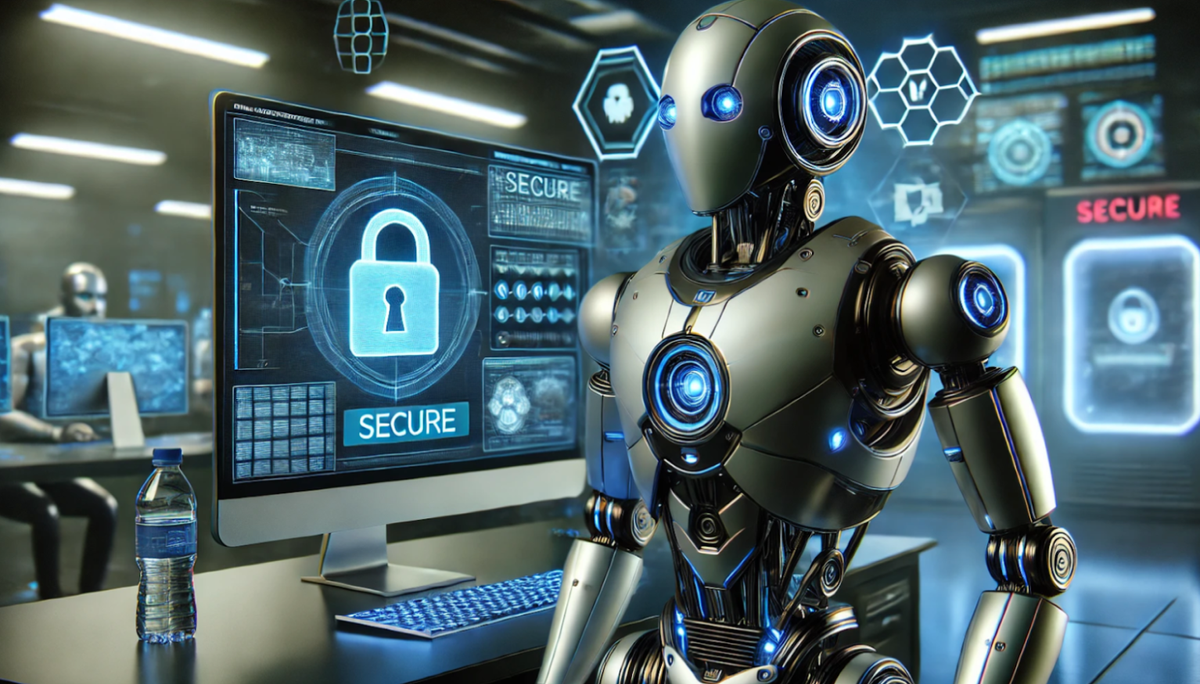
Introduction
The insurance industry is increasingly leveraging artificial intelligence (AI) to combat fraud, a pervasive issue that costs insurers billions of dollars annually. AI for fraud detection in insurance involves using advanced technologies such as machine learning, data analytics, and neural networks to identify, prevent, and mitigate fraudulent activities. This essay explores the key components, benefits, challenges, and future outlook of AI in fraud detection within the insurance sector.
Types of Fraud Committed in the Insurance Industry
Insurance fraud can take many forms, each with its own methods and implications. Understanding these types can help in comprehending the scope of the problem and the necessity of advanced detection methods.
- Application Fraud: This occurs when individuals or businesses provide false information when applying for insurance. Common examples include underreporting the value of property or assets, misrepresenting the expectations for future profit, or lying about one's past performance to obtain lower premiums.
- Claims Fraud: This involves false or exaggerated claims to receive compensation. Examples include staging car accidents, inflating repair costs, claiming non-existent injuries, or reporting thefts that did not occur. This type of fraud is one of the most significant challenges for insurers.
- False Denials and Underpayments: Insurers themselves can commit fraud by wrongfully denying legitimate claims or underpaying the amount due to policyholders. This fraudulent practice can damage the insurer's reputation and lead to legal consequences.
- Fictitious Payees: Creating fake entities or individuals to receive insurance payouts is another fraudulent method. These fake payees might be used in schemes involving life insurance, health insurance, or workers' compensation claims.
Key Components of AI in Fraud Detection
AI in fraud detection primarily utilizes machine learning algorithms to analyze patterns and detect anomalies in data. These algorithms can process vast amounts of historical and real-time data, learning to distinguish between legitimate and suspicious claims. One of the critical tools is supervised learning, where algorithms are trained on labeled datasets containing examples of both fraudulent and non-fraudulent claims. This training enables the system to identify similar patterns in new data. For instance, companies like Shift Technology offer AI-driven solutions that help insurers detect fraud by analyzing patterns in large datasets.
Another example includes The Hartford which uses a sophisticated AI model to detect fraudulent workers' compensation claims. The model analyzes data such as the timing of the claim, the nature of the injury, the claimant’s medical history, and workplace incident reports. By identifying patterns that are indicative of fraud, The Hartford can prioritize investigations and reduce fraudulent payouts.
Another essential component is unsupervised learning, which does not rely on labeled data. Instead, it identifies outliers and unusual patterns that deviate from the norm, flagging them for further investigation. This approach is particularly useful for detecting new types of fraud that may not have been previously identified. An example is SAS’s Fraud Framework which employs unsupervised learning to detect anomalous behaviors that signify potential fraud.
MetLife utilizes anomaly detection algorithms to spot unusual patterns in claims data. These algorithms can identify claims that significantly deviate from normal behavior, such as an unusually high number of claims from a single policyholder in a short period. MetLife’s AI system also integrates with their fraud investigation unit, providing real-time alerts for high-risk claims.
Additionally, natural language processing (NLP) is used to analyze textual data in claims forms and customer communications, detecting inconsistencies and suspicious language indicative of fraud. For example, Friss will use NLP to examine whether the claimant describes an incident using vague or contradictory language and then will use that to determine whether the claim should be flagged for a more detailed review.
Benefits of AI in Fraud Detection
The implementation of AI in fraud detection offers numerous benefits to the insurance industry. Firstly, it significantly enhances the accuracy and efficiency of fraud detection. Traditional methods rely heavily on manual reviews and rule-based systems, which can be time-consuming and prone to error. AI algorithms, on the other hand, can analyze vast datasets quickly and accurately, identifying complex patterns that humans might miss. According to a McKinsey report, AI can improve the accuracy of fraud detection by up to 50%.
Secondly, AI reduces the operational costs associated with fraud detection. Automated systems can handle a higher volume of claims with fewer resources, allowing human investigators to focus on the most complex and high-risk cases. This leads to more efficient use of personnel and a reduction in overall investigation costs. For example, FICO offers solutions that help insurers save millions by optimizing the fraud detection process.
Thirdly, AI improves the customer experience by speeding up the claims process. Legitimate claims can be processed more quickly when fraudulent claims are accurately identified and separated early in the process. This not only enhances customer satisfaction but also strengthens the insurer's reputation for reliability and efficiency. Companies like Lemonade have implemented AI to streamline the claims process, significantly reducing the time it takes to settle legitimate claims.
Challenges in Implementing AI for Fraud Detection
Despite its benefits, the implementation of AI for fraud detection in insurance is not without challenges. One of the primary concerns is data quality and availability. AI systems require large volumes of high-quality data to train and operate effectively. In many cases, data may be incomplete, inconsistent, or siloed across different systems, complicating the training and deployment of AI models. Accenture highlights the importance of integrating disparate data sources to create a unified dataset for effective AI implementation.
Furthermore, there are concerns related to data privacy and security. The use of AI in fraud detection involves processing sensitive customer information, which must be protected against breaches and unauthorized access. Insurers must implement stringent data security measures and comply with relevant regulations to safeguard customer data and maintain trust. The European Union's General Data Protection Regulation (GDPR) provides a framework for ensuring data protection and privacy in AI applications.
Conclusion
In conclusion, AI represents a powerful tool in the fight against insurance fraud. By enhancing the accuracy and efficiency of fraud detection, reducing operational costs, and improving the customer experience, AI offers significant benefits to the insurance industry. However, its implementation also presents challenges that must be carefully managed to ensure success. As technology continues to evolve, the insurance sector must adapt and innovate to fully leverage the potential of AI, paving the way for a more secure and efficient future in fraud detection.
Limit AI is here to revolutionize your workflow.
Limit has built the State of the Art AI for insurance. Limit AI will summarize and compare your quotes, run your surplus lines taxes and fee calculations, identify coverage deficiencies, and do what you need to get your job done. Limit AI is extremely well-versed in all lines of P&C and highly skilled at analyzing your policies & quotes.
Our AI Assistant is built on Limit’s years of expertise as a commercial insurance wholesaler with hands on experience in all lines of P&C. Limit AI answers questions, drafts emails, and compares quotes & policies with substantially more rigor and attention to nuance than any other competitive AI product today.
Ready to get started? Join the waitlist by visiting limit.com/ai or email us at contact@limit.com.